In a rubber duckie race there is usually a clear winner and a bunch that lag behind. But is there something special about the winning duck or is it just the variables of the stream?
The most recommended practice for ad testing in Meta is to have a campaign for test creatives and another campaign for scaling. The practice is to move winning creatives from the test campaign over to the scale campaign. However, in the new campaign the creative that once worked often fails. The reason it breaks down is what we call “the rubber duckie problem.”
Meta’s algorithm is smart. Really smart. But the sacrifice for using it is that it’s impossible to create identical A/B test conditions for creatives. Just like the duckies, ad creatives are at the mercy of the stream. If your creative is performing well in one campaign, relaunching it in a new campaign could have completely different results.
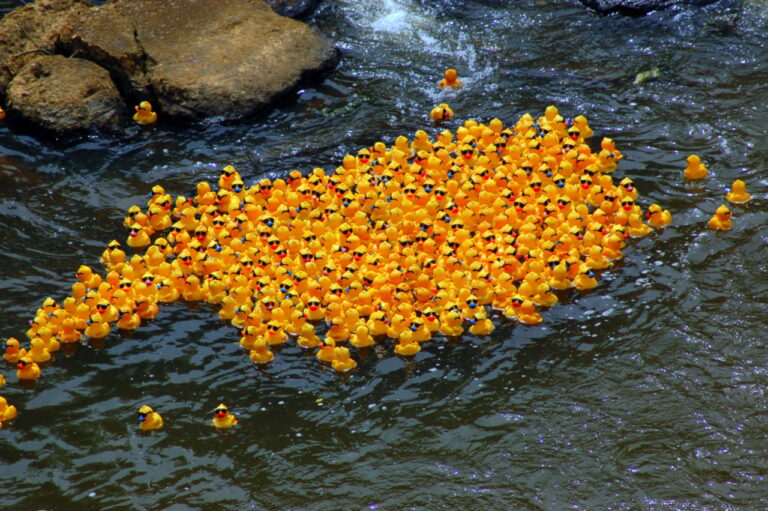
We know that the Meta algorithm works off of attention. Most likely it’s scroll speed plus other interactions. If you scroll over some objects more slowly the platform sees that as an attention signal. Take a few moments to look at a mattress ad and you’ll see more mattress ads.
We speculate the model adds a multiplier to early signals (learning mode). To drive ad revenue the algorithm needs to be ahead of the curve. Early interest will boost the placements of that creative. Boosted placements will drive more outcomes and a positive feedback cycle can take hold.
The flip side is also possible. Early bets means that creatives without early interactions will be penalized. The algorithm will add some drag to keep them out. This friction can be overcome with time, but all indications and metrics will make it look like a failed creative until such time as the statistical model picks up enough signal to boost it again.
The thing about early bets is Meta only needs to be right a slim majority of the time to drive more ad revenue. Ultimately, it’s good for advertisers because it drives outcomes much faster.
In practice we agonize about how much we can change a winning combination before it triggers a response from Meta’s model that would send it back to “learning” mode. We don’t move them to new campaigns and we don’t make tweaks to try to squeeze out a little bit more performance. Our recommendation for creative winners: go with the flow.